
Unlocking the Potential of Machine Learning in Health Economics ResearchHCHE Brownbag Seminar: Mona Aghdaee about Machine Learning in Healthcare Outcome Research
10 July 2024, 12:00 pm
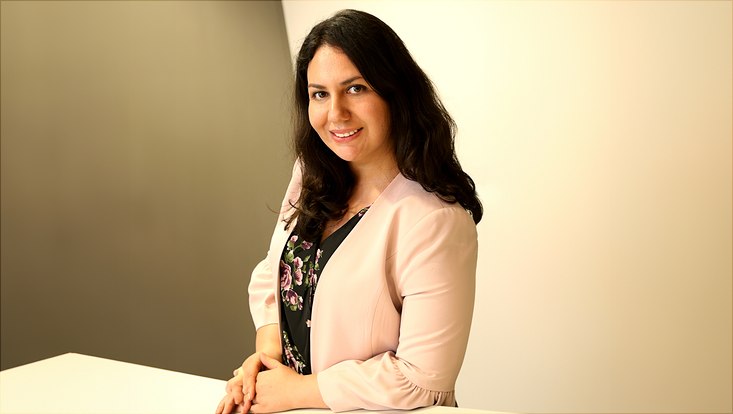
Photo: Mona Aghdaee
In their latest paper, researchers explore the world of Patient-Reported Outcome Measures (PROMs) and the critical role they play in health outcomes research. However, existing non-preference-based PROMs often fall short when it comes to estimating health state utilities, hindering their usefulness for economic evaluations. That's where this research comes in!
Mona Aghdaee from Macquarie University Sydney is researching a solution: mapping PROMs to a multi-attribute utility instrument using machine learning techniques. But why machine learning? Because traditional econometric methods sometimes fail to capture the complex interactions between variables, leading to inaccurate predictions and suboptimal funding decisions.
This study compares six machine learning techniques with eight econometric techniques, evaluating their efficacy in mapping. The results? The LASSO model outperformed all machine learning techniques, while the ALDVMM excelled among econometric methods. But the real magic happened when they combined the strengths of both in a hybrid model, offering promising insights for future health economics research.
Mona Aghdaee is a Research Fellow at the Macquarie University Centre for the Health Economy. Her academic background in mathematics and statistics has equipped her with a significant advantage in conducting research, particularly in the areas of policy analysis and modelling in health economics. Recently, she was admitted to the PhD program in Economics at the Macquarie Centre for the Health Economy, where she will be exploring the application of Artificial Intelligence in health economics, a subject that reflects her passion for innovative research.